Econometrics
The most authoritative and up-to-date core econometrics textbook available
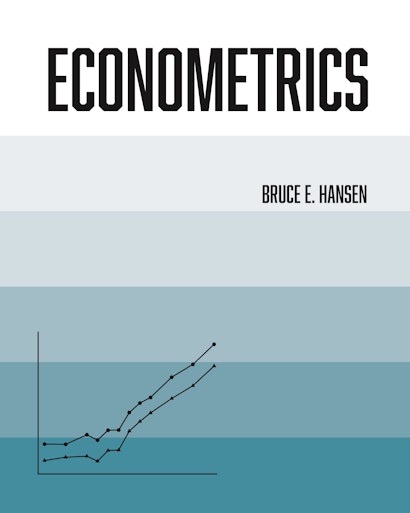
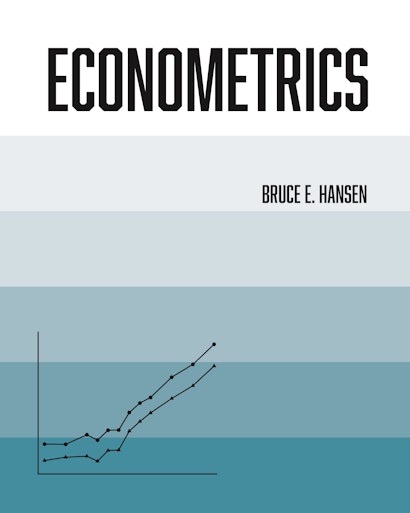
Hardcover
- Sale Price:
- $75.60/£63.00
- Price:
-
$108.00/£90.00 - ISBN:
- Published:
- Jun 28, 2022
- Copyright:
- 2022
- Illus:
- 167 b/w illus. 50 tables.
- Main_subject:
- Economics & Finance
30% off with code PUP30
ebook
- Sale Price:
- $75.60/£63.00
- Price:
-
$108.00/£90.00 - ISBN:
- Published:
- Jun 28, 2022
- Copyright:
- 2022
- Illus:
- 167 b/w illus. 50 tables.
- Main_subject:
- Economics & Finance
30% off with code PUP30
Econometrics is the quantitative language of economic theory, analysis, and empirical work, and it has become a cornerstone of graduate economics programs. Econometrics provides graduate and PhD students with an essential introduction to this foundational subject in economics and serves as an invaluable reference for researchers and practitioners. This comprehensive textbook teaches fundamental concepts, emphasizes modern, real-world applications, and gives students an intuitive understanding of econometrics.
- Covers the full breadth of econometric theory and methods with mathematical rigor while emphasizing intuitive explanations that are accessible to students of all backgrounds
- Draws on integrated, research-level datasets, provided on an accompanying website
- Discusses linear econometrics, time series, panel data, nonparametric methods, nonlinear econometric models, and modern machine learning
- Features hundreds of exercises that enable students to learn by doing
- Includes in-depth appendices on matrix algebra and useful inequalities and a wealth of real-world examples
- Can serve as a core textbook for a first-year PhD course in econometrics and as a follow-up to Bruce E. Hansen’s Probability and Statistics for Economists
- Preface
- Acknowledgments
- Notation
- 1 Introduction
- 1.1 What Is Econometrics?
- 1.2 The Probability Approach to Econometrics
- 1.3 Econometric Terms
- 1.4 Observational Data
- 1.5 Standard Data Structures
- 1.6 Econometric Software
- 1.7 Replication
- 1.8 Data Files for Textbook
- 1.9 Reading the Book
- I Regression
- 2 Conditional Expectation and Projection
- 2.1 Introduction
- 2.2 The Distribution of Wages
- 2.3 Conditional Expectation
- 2.4 Logs and Percentages
- 2.5 Conditional Expectation Function
- 2.6 Continuous Variables
- 2.7 Law of Iterated Expectations
- 2.8 CEF Error
- 2.9 Intercept-Only Model
- 2.10 Regression Variance
- 2.11 Best Predictor
- 2.12 Conditional Variance
- 2.13 Homoskedasticity and Heteroskedasticity
- 2.14 Regression Derivative
- 2.15 Linear CEF
- 2.16 Linear CEF with Nonlinear Effects
- 2.17 Linear CEF with Dummy Variables
- 2.18 Best Linear Predictor
- 2.19 Illustrations of Best Linear Predictor
- 2.20 Linear Predictor Error Variance
- 2.21 Regression Coefficients
- 2.22 Regression Subvectors
- 2.23 Coefficient Decomposition
- 2.24 Omitted Variable Bias
- 2.25 Best Linear Approximation
- 2.26 Regression to the Mean
- 2.27 Reverse Regression
- 2.28 Limitations of the Best Linear Projection
- 2.29 Random Coefficient Model
- 2.30 Causal Effects
- 2.31 Existence and Uniqueness of the Conditional Expectation*
- 2.32 Identification*
- 2.33 Technical Proofs*
- 2.34 Exercises
- 3 The Algebra of Least Squares
- 3.1 Introduction
- 3.2 Samples
- 3.3 Moment Estimators
- 3.4 Least Squares Estimator
- 3.5 Solving for Least Squares with One Regressor
- 3.6 Solving for Least Squares with Multiple Regressors
- 3.7 Illustration
- 3.8 Least Squares Residuals
- 3.9 Demeaned Regressors
- 3.10 Model in Matrix Notation
- 3.11 Projection Matrix
- 3.12 Annihilator Matrix
- 3.13 Estimation of Error Variance
- 3.14 Analysis of Variance
- 3.15 Projections
- 3.16 Regression Components
- 3.17 Regression Components (Alternative Derivation)*
- 3.18 Residual Regression
- 3.19 Leverage Values
- 3.20 Leave-One-Out Regression
- 3.21 Influential Observations
- 3.22 CPS Dataset
- 3.23 Numerical Computation
- 3.24 Collinearity Errors
- 3.25 Programming
- 3.26 Exercises
- 4 Least Squares Regression
- 4.1 Introduction
- 4.2 Random Sampling
- 4.3 Sample Mean
- 4.4 Linear Regression Model
- 4.5 Expectation of Least Squares Estimator
- 4.6 Variance of Least Squares Estimator
- 4.7 Unconditional Moments
- 4.8 Gauss-Markov Theorem
- 4.9 Generalized Least Squares
- 4.10 Residuals
- 4.11 Estimation of Error Variance
- 4.12 Mean-Squared Forecast Error
- 4.13 Covariance Matrix Estimation under Homoskedasticity
- 4.14 Covariance Matrix Estimation under Heteroskedasticity
- 4.15 Standard Errors
- 4.16 Estimation with Sparse Dummy Variables
- 4.17 Computation
- 4.18 Measures of Fit
- 4.19 Empirical Example
- 4.20 Multicollinearity
- 4.21 Clustered Sampling
- 4.22 Inference with Clustered Samples
- 4.23 At What Level to Cluster?
- 4.24 Technical Proofs*
- 4.25 Exercises
- 5 Normal Regression
- 5.1 Introduction
- 5.2 The Normal Distribution
- 5.3 Multivariate Normal Distribution
- 5.4 Joint Normality and Linear Regression
- 5.5 Normal Regression Model
- 5.6 Distribution of OLS Coefficient Vector
- 5.7 Distribution of OLS Residual Vector
- 5.8 Distribution of Variance Estimator
- 5.9 t-Statistic
- 5.10 Confidence Intervals for Regression Coefficients
- 5.11 Confidence Intervals for Error Variance
- 5.12 t-Test
- 5.13 Likelihood Ratio Test
- 5.14 Information Bound for Normal Regression
- 5.15 Exercises
- 2 Conditional Expectation and Projection
- II Large Sample Methods
- 6 A Review of Large Sample Asymptotics
- 6.1 Introduction
- 6.2 Modes of Convergence
- 6.3 Weak Law of Large Numbers
- 6.4 Central Limit Theorem
- 6.5 Continuous Mapping Theorem and Delta Method
- 6.6 Smooth Function Model
- 6.7 Stochastic Order Symbols
- 6.8 Convergence of Moments
- 7 Asymptotic Theory for Least Squares
- 7.1 Introduction
- 7.2 Consistency of Least Squares Estimator
- 7.3 Asymptotic Normality
- 7.4 Joint Distribution
- 7.5 Consistency of Error Variance Estimators
- 7.6 Homoskedastic Covariance Matrix Estimation
- 7.7 Heteroskedastic Covariance Matrix Estimation
- 7.8 Summary of Covariance Matrix Notation
- 7.9 Alternative Covariance Matrix Estimators*
- 7.10 Functions of Parameters
- 7.11 Asymptotic Standard Errors
- 7.12 t-Statistic
- 7.13 Confidence Intervals
- 7.14 Regression Intervals
- 7.15 Forecast Intervals
- 7.16 Wald Statistic
- 7.17 Homoskedastic Wald Statistic
- 7.18 Confidence Regions
- 7.19 Edgeworth Expansion*
- 7.20 Uniformly Consistent Residuals*
- 7.21 Asymptotic Leverage*
- 7.22 Exercises
- 8 Restricted Estimation
- 8.1 Introduction
- 8.2 Constrained Least Squares
- 8.3 Exclusion Restriction
- 8.4 Finite Sample Properties
- 8.5 Minimum Distance
- 8.6 Asymptotic Distribution
- 8.7 Variance Estimation and Standard Errors
- 8.8 Efficient Minimum Distance Estimator
- 8.9 Exclusion Restriction Revisited
- 8.10 Variance and Standard Error Estimation
- 8.11 Hausman Equality
- 8.12 Example: Mankiw, Romer, and Weil (1992)
- 8.13 Misspecification
- 8.14 Nonlinear Constraints
- 8.15 Inequality Restrictions
- 8.16 Technical Proofs*
- 8.17 Exercises
- 9 Hypothesis Testing
- 9.1 Introduction
- 9.2 Hypotheses
- 9.3 Acceptance and Rejection
- 9.4 Type I Error
- 9.5 T-Tests
- 9.6 Type II Error and Power
- 9.7 Statistical Significance
- 9.8 p-Values
- 9.9 t-Ratios and the Abuse of Testing
- 9.10 Wald Tests
- 9.11 Homoskedastic Wald Tests
- 9.12 Criterion-Based Tests
- 9.13 Minimum Distance Tests
- 9.14 Minimum Distance Tests under Homoskedasticity
- 9.15 F Tests
- 9.16 Hausman Tests
- 9.17 Score Tests
- 9.18 Problems with Tests of Nonlinear Hypotheses
- 9.19 Monte Carlo Simulation
- 9.20 Confidence Intervals by Test Inversion
- 9.21 Multiple Tests and Bonferroni Corrections
- 9.22 Power and Test Consistency
- 9.23 Asymptotic Local Power
- 9.24 Asymptotic Local Power, Vector Case
- 9.25 Exercises
- 10 Resampling Methods
- 10.1 Introduction
- 10.2 Example
- 10.3 Jackknife Estimation of Variance
- 10.4 Example
- 10.5 Jackknife for Clustered Observations
- 10.6 The Bootstrap Algorithm
- 10.7 Bootstrap Variance and Standard Errors
- 10.8 Percentile Interval
- 10.9 The Bootstrap Distribution
- 10.10 The Distribution of the Bootstrap Observations
- 10.11 The Distribution of the Bootstrap Sample Mean
- 10.12 Bootstrap Asymptotics
- 10.13 Consistency of the Bootstrap Estimate of Variance
- 10.14 Trimmed Estimator of Bootstrap Variance
- 10.15 Unreliability of Untrimmed Bootstrap Standard Errors
- 10.16 Consistency of the Percentile Interval
- 10.17 Bias-Corrected Percentile Interval
- 10.18 BCa Percentile Interval
- 10.19 Percentile-t Interval
- 10.20 Percentile-t Asymptotic Refinement
- 10.21 Bootstrap Hypothesis Tests
- 10.22 Wald-Type Bootstrap Tests
- 10.23 Criterion-Based Bootstrap Tests
- 10.24 Parametric Bootstrap
- 10.25 How Many Bootstrap Replications?
- 10.26 Setting the Bootstrap Seed
- 10.27 Bootstrap Regression
- 10.28 Bootstrap Regression Asymptotic Theory
- 10.29 Wild Bootstrap
- 10.30 Bootstrap for Clustered Observations
- 10.31 Technical Proofs*
- 10.32 Exercises
- 6 A Review of Large Sample Asymptotics
- III Multiple Equation Models
- 11 Multivariate Regression
- 11.1 Introduction
- 11.2 Regression Systems
- 11.3 Least Squares Estimator
- 11.4 Expectation and Variance of Systems Least Squares
- 11.5 Asymptotic Distribution
- 11.6 Covariance Matrix Estimation
- 11.7 Seemingly Unrelated Regression
- 11.8 Equivalence of SUR and Least Squares
- 11.9 Maximum Likelihood Estimator
- 11.10 Restricted Estimation
- 11.11 Reduced Rank Regression
- 11.12 Principal Component Analysis
- 11.13 Factor Models
- 11.14 Approximate Factor Models
- 11.15 Factor Models with Additional Regressors
- 11.16 Factor-Augmented Regression
- 11.17 Multivariate Normal*
- 11.18 Exercises
- 12 Instrumental Variables
- 12.1 Introduction
- 12.2 Overview
- 12.3 Examples
- 12.4 Endogenous Regressors
- 12.5 Instruments
- 12.6 Example: College Proximity
- 12.7 Reduced Form
- 12.8 Identification
- 12.9 Instrumental Variables Estimator
- 12.10 Demeaned Representation
- 12.11 Wald Estimator
- 12.12 Two-Stage Least Squares
- 12.13 Limited Information Maximum Likelihood
- 12.14 Split-Sample IV and JIVE
- 12.15 Consistency of 2SLS
- 12.16 Asymptotic Distribution of 2SLS
- 12.17 Determinants of 2SLS Variance
- 12.18 Covariance Matrix Estimation
- 12.19 LIML Asymptotic Distribution
- 12.20 Functions of Parameters
- 12.21 Hypothesis Tests
- 12.22 Finite Sample Theory
- 12.23 Bootstrap for 2SLS
- 12.24 The Peril of Bootstrap 2SLS Standard Errors
- 12.25 Clustered Dependence
- 12.26 Generated Regressors
- 12.27 Regression with Expectation Errors
- 12.28 Control Function Regression
- 12.29 Endogeneity Tests
- 12.30 Subset Endogeneity Tests
- 12.31 Overidentification Tests
- 12.32 Subset Overidentification Tests
- 12.33 Bootstrap Overidentification Tests
- 12.34 Local Average Treatment Effects
- 12.35 Identification Failure
- 12.36 Weak Instruments
- 12.37 Many Instruments
- 12.38 Testing for Weak Instruments
- 12.39 Weak Instruments with k2 > 1
- 12.40 Example: Acemoglu, Johnson, and Robinson (2001)
- 12.41 Example: Angrist and Krueger (1991)
- 12.42 Programming
- 12.43 Exercises
- 13 Generalized Method of Moments
- 13.1 Introduction
- 13.2 Moment Equation Models
- 13.3 Method of Moments Estimators
- 13.4 Overidentified Moment Equations
- 13.5 Linear Moment Models
- 13.6 GMM Estimator
- 13.7 Distribution of GMM Estimator
- 13.8 Efficient GMM
- 13.9 Efficient GMM versus 2SLS
- 13.10 Estimation of the Efficient Weight Matrix
- 13.11 Iterated GMM
- 13.12 Covariance Matrix Estimation
- 13.13 Clustered Dependence
- 13.14 Wald Test
- 13.15 Restricted GMM
- 13.16 Nonlinear Restricted GMM
- 13.17 Constrained Regression
- 13.18 Multivariate Regression
- 13.19 Distance Test
- 13.20 Continuously Updated GMM
- 13.21 Overidentification Test
- 13.22 Subset Overidentification Tests
- 13.23 Endogeneity Test
- 13.24 Subset Endogeneity Test
- 13.25 Nonlinear GMM
- 13.26 Bootstrap for GMM
- 13.27 Conditional Moment Equation Models
- 13.28 Technical Proofs*
- 13.29 Exercises
- 11 Multivariate Regression
- IV Dependent and Panel Data
- 14 Time Series
- 14.1 Introduction
- 14.2 Examples
- 14.3 Differences and Growth Rates
- 14.4 Stationarity
- 14.5 Transformations of Stationary Processes
- 14.6 Convergent Series
- 14.7 Ergodicity
- 14.8 Ergodic Theorem
- 14.9 Conditioning on Information Sets
- 14.10 Martingale Difference Sequences
- 14.11 CLT for Martingale Differences
- 14.12 Mixing
- 14.13 CLT for Correlated Observations
- 14.14 Linear Projection
- 14.15 White Noise
- 14.16 The Wold Decomposition
- 14.17 Lag Operator
- 14.18 Autoregressive Wold Representation
- 14.19 Linear Models
- 14.20 Moving Average Process
- 14.21 Infinite-Order Moving Average Process
- 14.22 First-Order Autoregressive Process
- 14.23 Unit Root and Explosive AR(1) Processes
- 14.24 Second-Order Autoregressive Process
- 14.25 AR(p) Process
- 14.26 Impulse Response Function
- 14.27 ARMA and ARIMA Processes
- 14.28 Mixing Properties of Linear Processes
- 14.29 Identification
- 14.30 Estimation of Autoregressive Models
- 14.31 Asymptotic Distribution of Least Squares Estimator
- 14.32 Distribution under Homoskedasticity
- 14.33 Asymptotic Distribution under General Dependence
- 14.34 Covariance Matrix Estimation
- 14.35 Covariance Matrix Estimation under General Dependence
- 14.36 Testing the Hypothesis of No Serial Correlation
- 14.37 Testing for Omitted Serial Correlation
- 14.38 Model Selection
- 14.39 Illustrations
- 14.40 Time Series Regression Models
- 14.41 Static, Distributed Lag, and Autoregressive Distributed Lag Models
- 14.42 Time Trends
- 14.43 Illustration
- 14.44 Granger Causality
- 14.45 Testing for Serial Correlation in Regression Models
- 14.46 Bootstrap for Time Series
- 14.47 Technical Proofs*
- 14.48 Exercises
- 15 Multivariate Time Series
- 15.1 Introduction
- 15.2 Multiple Equation Time Series Models
- 15.3 Linear Projection
- 15.4 Multivariate Wold Decomposition
- 15.5 Impulse Response
- 15.6 VAR(1) Model
- 15.7 VAR(p) Model
- 15.8 Regression Notation
- 15.9 Estimation
- 15.10 Asymptotic Distribution
- 15.11 Covariance Matrix Estimation
- 15.12 Selection of Lag Length in a VAR
- 15.13 Illustration
- 15.14 Predictive Regressions
- 15.15 Impulse Response Estimation
- 15.16 Local Projection Estimator
- 15.17 Regression on Residuals
- 15.18 Orthogonalized Shocks
- 15.19 Orthogonalized Impulse Response Function
- 15.20 Orthogonalized Impulse Response Estimation
- 15.21 Illustration
- 15.22 Forecast Error Decomposition
- 15.23 Identification of Recursive VARs
- 15.24 Oil Price Shocks
- 15.25 Structural VARs
- 15.26 Identification of Structural VARs
- 15.27 Long-Run Restrictions
- 15.28 Blanchard and Quah (1989) Illustration
- 15.29 External Instruments
- 15.30 Dynamic Factor Models
- 15.31 Technical Proofs*
- 15.32 Exercises
- 16 Nonstationary Time Series
- 16.1 Introduction
- 16.2 Partial Sum Process and Functional Convergence
- 16.3 Beveridge-Nelson Decomposition
- 16.4 Functional CLT
- 16.5 Orders of Integration
- 16.6 Means, Local Means, and Trends
- 16.7 Demeaning and Detrending
- 16.8 Stochastic Integrals
- 16.9 Estimation of an AR(1)
- 16.10 AR(1) Estimation with an Intercept
- 16.11 Sample Covariances of Integrated and Stationary Processes
- 16.12 AR(p) Models with a Unit Root
- 16.13 Testing for a Unit Root
- 16.14 KPSS Stationarity Test
- 16.15 Spurious Regression
- 16.16 Nonstationary VARs
- 16.17 Cointegration
- 16.18 Role of Intercept and Trend
- 16.19 Cointegrating Regression
- 16.20 VECM Estimation
- 16.21 Testing for Cointegration in a VECM
- 16.22 Technical Proofs*
- 16.23 Exercises
- 17 Panel Data
- 17.1 Introduction
- 17.2 Time Indexing and Unbalanced Panels
- 17.3 Notation
- 17.4 Pooled Regression
- 17.5 One-Way Error Component Model
- 17.6 Random Effects
- 17.7 Fixed Effects Model
- 17.8 Within Transformation
- 17.9 Fixed Effects Estimator
- 17.10 Differenced Estimator
- 17.11 Dummy Variables Regression
- 17.12 Fixed Effects Covariance Matrix Estimation
- 17.13 Fixed Effects Estimation in Stata
- 17.14 Between Estimator
- 17.15 Feasible GLS
- 17.16 Intercept in Fixed Effects Regression
- 17.17 Estimation of Fixed Effects
- 17.18 GMM Interpretation of Fixed Effects
- 17.19 Identification in the Fixed Effects Model
- 17.20 Asymptotic Distribution of Fixed Effects Estimator
- 17.21 Asymptotic Distribution for Unbalanced Panels
- 17.22 Heteroskedasticity-Robust Covariance Matrix Estimation
- 17.23 Heteroskedasticity-Robust Estimation—Unbalanced Case
- 17.24 Hausman Test for Random vs. Fixed Effects
- 17.25 Random Effects or Fixed Effects?
- 17.26 Time Trends
- 17.27 Two-Way Error Components
- 17.28 Instrumental Variables
- 17.29 Identification with Instrumental Variables
- 17.30 Asymptotic Distribution of Fixed Effects 2SLS Estimator
- 17.31 Linear GMM
- 17.32 Estimation with Time-Invariant Regressors
- 17.33 Hausman-Taylor Model
- 17.34 Jackknife Covariance Matrix Estimation
- 17.35 Panel Bootstrap
- 17.36 Dynamic Panel Models
- 17.37 The Bias of Fixed Effects Estimation
- 17.38 Anderson-Hsiao Estimator
- 17.39 Arellano-Bond Estimator
- 17.40 Weak Instruments
- 17.41 Dynamic Panels with Predetermined Regressors
- 17.42 Blundell-Bond Estimator
- 17.43 Forward Orthogonal Transformation
- 17.44 Empirical Illustration
- 17.45 Exercises
- 18 Difference in Differences
- 18.1 Introduction
- 18.2 Minimum Wage in New Jersey
- 18.3 Identification
- 18.4 Multiple Units
- 18.5 Do Police Reduce Crime?
- 18.6 Trend Specification
- 18.7 Do Blue Laws Affect Liquor Sales?
- 18.8 Check Your Code: Does Abortion Impact Crime?
- 18.9 Inference
- 18.10 Exercises
- 14 Time Series
- V Nonparametric Methods
- 19 Nonparametric Regression
- 19.1 Introduction
- 19.2 Binned Means Estimator
- 19.3 Kernel Regression
- 19.4 Local Linear Estimator
- 19.5 Local Polynomial Estimator
- 19.6 Asymptotic Bias
- 19.7 Asymptotic Variance
- 19.8 AIMSE
- 19.9 Reference Bandwidth
- 19.10 Estimation at a Boundary
- 19.11 Nonparametric Residuals and Prediction Errors
- 19.12 Cross-Validation Bandwidth Selection
- 19.13 Asymptotic Distribution
- 19.14 Undersmoothing
- 19.15 Conditional Variance Estimation
- 19.16 Variance Estimation and Standard Errors
- 19.17 Confidence Bands
- 19.18 The Local Nature of Kernel Regression
- 19.19 Application to Wage Regression
- 19.20 Clustered Observations
- 19.21 Application to Test Scores
- 19.22 Multiple Regressors
- 19.23 Curse of Dimensionality
- 19.24 Partially Linear Regression
- 19.25 Computation
- 19.26 Technical Proofs*
- 19.27 Exercises
- 20 Series Regression
- 20.1 Introduction
- 20.2 Polynomial Regression
- 20.3 Illustrating Polynomial Regression
- 20.4 Orthogonal Polynomials
- 20.5 Splines
- 20.6 Illustrating Spline Regression
- 20.7 The Global/Local Nature of Series Regression
- 20.8 Stone-Weierstrass and Jackson Approximation Theory
- 20.9 Regressor Bounds
- 20.10 Matrix Convergence
- 20.11 Consistent Estimation
- 20.12 Convergence Rate
- 20.13 Asymptotic Normality
- 20.14 Regression Estimation
- 20.15 Undersmoothing
- 20.16 Residuals and Regression Fit
- 20.17 Cross-Validation Model Selection
- 20.18 Variance and Standard Error Estimation
- 20.19 Clustered Observations
- 20.20 Confidence Bands
- 20.21 Uniform Approximations
- 20.22 Partially Linear Model
- 20.23 Panel Fixed Effects
- 20.24 Multiple Regressors
- 20.25 Additively Separable Models
- 20.26 Nonparametric Instrumental Variables Regression
- 20.27 NPIV Identification
- 20.28 NPIV Convergence Rate
- 20.29 Nonparametric vs. Parametric Identification
- 20.30 Example: Angrist and Lavy (1999)
- 20.31 Technical Proofs*
- 20.32 Exercises
- 21 Regression Discontinuity
- 21.1 Introduction
- 21.2 Sharp Regression Discontinuity
- 21.3 Identification
- 21.4 Estimation
- 21.5 Inference
- 21.6 Bandwidth Selection
- 21.7 RDD with Covariates
- 21.8 A Simple RDD Estimator
- 21.9 Density Discontinuity Test
- 21.10 Fuzzy Regression Discontinuity
- 21.11 Estimation of FRD
- 21.12 Exercises
- 19 Nonparametric Regression
- VI Nonlinear Methods
- 22 M-Estimators
- 22.1 Introduction
- 22.2 Examples
- 22.3 Identification and Estimation
- 22.4 Consistency
- 22.5 Uniform Law of Large Numbers
- 22.6 Asymptotic Distribution
- 22.7 Asymptotic Distribution under Broader Conditions*
- 22.8 Covariance Matrix Estimation
- 22.9 Technical Proofs*
- 22.10 Exercises
- 23 Nonlinear Least Squares
- 23.1 Introduction
- 23.2 Identification
- 23.3 Estimation
- 23.4 Asymptotic Distribution
- 23.5 Covariance Matrix Estimation
- 23.6 Panel Data
- 23.7 Threshold Models
- 23.8 Testing for Nonlinear Components
- 23.9 Computation
- 23.10 Technical Proofs*
- 23.11 Exercises
- 24 Quantile Regression
- 24.1 Introduction
- 24.2 Median Regression
- 24.3 Least Absolute Deviations
- 24.4 Quantile Regression
- 24.5 Example Quantile Shapes
- 24.6 Estimation
- 24.7 Asymptotic Distribution
- 24.8 Covariance Matrix Estimation
- 24.9 Clustered Dependence
- 24.10 Quantile Crossings
- 24.11 Quantile Causal Effects
- 24.12 Random Coefficient Representation
- 24.13 Nonparametric Quantile Regression
- 24.14 Panel Data
- 24.15 IV Quantile Regression
- 24.16 Technical Proofs*
- 24.17 Exercises
- 25 Binary Choice
- 25.1 Introduction
- 25.2 Binary Choice Models
- 25.3 Models for the Response Probability
- 25.4 Latent Variable Interpretation
- 25.5 Likelihood
- 25.6 Pseudo-True Values
- 25.7 Asymptotic Distribution
- 25.8 Covariance Matrix Estimation
- 25.9 Marginal Effects
- 25.10 Application
- 25.11 Semiparametric Binary Choice
- 25.12 IV Probit
- 25.13 Binary Panel Data
- 25.14 Technical Proofs*
- 25.15 Exercises
- 26 Multiple Choice
- 26.1 Introduction
- 26.2 Multinomial Response
- 26.3 Multinomial Logit
- 26.4 Conditional Logit
- 26.5 Independence of Irrelevant Alternatives
- 26.6 Nested Logit
- 26.7 Mixed Logit
- 26.8 Simple Multinomial Probit
- 26.9 General Multinomial Probit
- 26.10 Ordered Response
- 26.11 Count Data
- 26.12 BLP Demand Model
- 26.13 Technical Proofs*
- 26.14 Exercises
- 27 Censoring and Selection
- 27.1 Introduction
- 27.2 Censoring
- 27.3 Censored Regression Functions
- 27.4 The Bias of Least Squares Estimation
- 27.5 Tobit Estimator
- 27.6 Identification in Tobit Regression
- 27.7 CLAD and CQR Estimators
- 27.8 Illustrating Censored Regression
- 27.9 Sample Selection Bias
- 27.10 Heckman’s Model
- 27.11 Nonparametric Selection
- 27.12 Panel Data
- 27.13 Exercises
- 28 Model Selection, Stein Shrinkage, and Model Averaging
- 28.1 Introduction
- 28.2 Model Selection
- 28.3 Bayesian Information Criterion
- 28.4 Akaike Information Criterion for Regression
- 28.5 Akaike Information Criterion for Likelihood
- 28.6 Mallows Criterion
- 28.7 Hold-Out Criterion
- 28.8 Cross-Validation Criterion
- 28.9 K-Fold Cross-Validation
- 28.10 Many Selection Criteria Are Similar
- 28.11 Relation with Likelihood Ratio Testing
- 28.12 Consistent Selection
- 28.13 Asymptotic Selection Optimality
- 28.14 Focused Information Criterion
- 28.15 Best Subset and Stepwise Regression
- 28.16 The MSE of Model Selection Estimators
- 28.17 Inference after Model Selection
- 28.18 Empirical Illustration
- 28.19 Shrinkage Methods
- 28.20 James-Stein Shrinkage Estimator
- 28.21 Interpretation of the Stein Effect
- 28.22 Positive Part Estimator
- 28.23 Shrinkage Toward Restrictions
- 28.24 Group James-Stein
- 28.25 Empirical Illustrations
- 28.26 Model Averaging
- 28.27 Smoothed BIC and AIC
- 28.28 Mallows Model Averaging
- 28.29 Jackknife (CV) Model Averaging
- 28.30 Granger-Ramanathan Averaging
- 28.31 Empirical Illustration
- 28.32 Technical Proofs*
- 28.33 Exercises
- 29 Machine Learning
- 29.1 Introduction
- 29.2 Big Data, High Dimensionality, and Machine Learning
- 29.3 High-Dimensional Regression
- 29.4 p-norms
- 29.5 Ridge Regression
- 29.6 Statistical Properties of Ridge Regression
- 29.7 Illustrating Ridge Regression
- 29.8 Lasso
- 29.9 Lasso Penalty Selection
- 29.10 Lasso Computation
- 29.11 Asymptotic Theory for the Lasso
- 29.12 Approximate Sparsity
- 29.13 Elastic Net
- 29.14 Post-Lasso
- 29.15 Regression Trees
- 29.16 Bagging
- 29.17 Random Forests
- 29.18 Ensembling
- 29.19 Lasso IV
- 29.20 Double Selection Lasso
- 29.21 Post-Regularization Lasso
- 29.22 Double/Debiased Machine Learning
- 29.23 Technical Proofs*
- 29.24 Exercises
- 22 M-Estimators
- Appendixes
- A Matrix Algebra
- A.1 Notation
- A.2 Complex Matrices
- A.3 Matrix Addition
- A.4 Matrix Multiplication
- A.5 Trace
- A.6 Rank and Inverse
- A.7 Orthogonal and Orthonormal Matrices
- A.8 Determinant
- A.9 Eigenvalues
- A.10 Positive Definite Matrices
- A.11 Idempotent Matrices
- A.12 Singular Values
- A.13 Matrix Decompositions
- A.14 Generalized Eigenvalues
- A.15 Extrema of Quadratic Forms
- A.16 Cholesky Decomposition
- A.17 QR Decomposition
- A.18 Solving Linear Systems
- A.19 Algorithmic Matrix Inversion
- A.20 Matrix Calculus
- A.21 Kronecker Products and the Vec Operator
- A.22 Vector Norms
- A.23 Matrix Norms
- B Useful-Inequalities
- B.1-Inequalities for Real Numbers
- B.2-Inequalities for Vectors
- B.3-Inequalities for Matrices
- B.4-Probability Inequalities
- B.5-Proofs*
- References
- Index